Plots Julia Contour
A simple line plot of the columns.
Plot function pair (x(u), y(u)).
- Created by Tom Breloff (@tbreloff) Maintained by the JuliaPlots members. Plots is a plotting API and toolset. My goals with the package are: Powerful. Do more with less. Complex visualizations become easy. Stop reading so much documentation. Commands should 'just work'. Less code means fewer mistakes and more.
- Julia 1.0.0でインストールする際に少し詰まった部分があったので、そこを解消する方法を記します。 インストール.
- Plots - powerful convenience for visualization in Julia. Author: Thomas Breloff (@tbreloff) To get started, see the tutorial. Almost everything in Plots is done by specifying plot attributes. Tap into the extensive visualization functionality enabled by the Plots ecosystem, and easily build your own complex graphics components with recipes.
Access predefined palettes (or build your own with the colorscheme
method). Line/marker colors are auto-generated from the plot's palette, unless overridden. Set the z
argument to turn on series gradients.
Change the guides/background/limits/ticks. Convenience args xaxis
and yaxis
allow you to pass a tuple or value which will be mapped to the relevant args automatically. The xaxis
below will be replaced with xlabel
and xlims
args automatically during the preprocessing step. You can also use shorthand functions: title!
, xaxis!
, yaxis!
, xlabel!
, ylabel!
, xlims!
, ylims!
, xticks!
, yticks!
Plot an image. y-axis is set to flipped
Plot multiple series with different numbers of points. Mix arguments that apply to all series (marker/markersize) with arguments unique to each series (colors). Special arguments line
, marker
, and fill
will automatically figure out what arguments to set (for example, we are setting the linestyle
, linewidth
, and color
arguments with line
.) Note that we pass a matrix of colors, and this applies the colors to each series.
Start with a base plot...
I want to plot contour lines for specific level values! As far as I know, you can say how many levels you want plotted using Plots pyplot V (x,y) = x ^2 + y ^2 x = linspace (-10, 10, 50) y = linspace (-10, 10, 50) contour (x,y, (x,y) -V (x,y), levels =30).
and add to it later.
x
is the midpoint of the bar. (todo: allow passing of edges instead of midpoints)
Use the layout
keyword, and optionally the convenient @layout
macro to generate arbitrarily complex subplot layouts.
Note here the automatic grid layout, as well as the order in which new series are added to the plots.
Create an OHLC chart. Pass in a list of (open,high,low,close) tuples as your y
argument. This uses recipes to first convert the tuples to OHLC objects, and subsequently create a :path series with the appropriate line segments.
The annotations
keyword is used for text annotations in data-coordinates. Pass in a tuple (x,y,text) or a vector of annotations. annotate!(ann)
is shorthand for plot!(; annotation=ann)
. Series annotations are used for annotating individual data points. They require only the annotation... x/y values are computed. A PlotText
object can be build with the method text(string, attr...)
, which wraps font and color attributes.
Any value for fill works here. We first build a filled contour from a function, then an unfilled contour from a matrix.
For a matrix mat
with unique nonzeros spy(mat)
returns a colorless plot. If mat
has various different nonzero values, a colorbar is added. The colorbar can be disabled with legend = nothing
.
The grid lines can be modified individually for each axis with the magic grid
argument.
The style of the frame/axes of a (sub)plot can be changed with the framestyle
attribute. The default framestyle is :axes
.
You can use the line_z
and marker_z
properties to associate a color with each line segment or marker in the plot.
see: http://stackoverflow.com/a/37732384/5075246
Ribbons can be added to lines via the ribbon
keyword; you can pass a tuple of arrays (upper and lower bounds), a single Array (for symmetric ribbons), a Function, or a number.
Missing values and non-finite values, including NaN
, are not plotted. Instead, lines are separated into segments at these values.
A lens lets you easily magnify a region of a plot. x and y coordinates refer to the to be magnified region and the via the inset
keyword the subplot index and the bounding box (in relative coordinates) of the inset plot with the magnified plot can be specified. Additional attributes count for the inset plot.
Plots supports different Array
types that follow the AbstractArray
interface, like StaticArrays
and OffsetArrays.
Allows to plot arbitrary 3d meshes. If only x,y,z are given the mesh is generated automatically. You can also specify the connections using the connections keyword. This is only supported on the Plotly backend. The connections are specified using a tuple of vectors. Each vector contains the 0-based indices of one point of a triangle, such that elements at the same position of these vectors form a triangle.
- Supported arguments:
annotations
,aspect_ratio
,background_color
,background_color_inside
,background_color_legend
,background_color_outside
,background_color_subplot
,bar_width
,bins
,bottom_margin
,camera
,clims
,color_palette
,colorbar
,colorbar_entry
,colorbar_title
,contour_labels
,discrete_values
,fill_z
,fillalpha
,fillcolor
,fillrange
,flip
,foreground_color
,foreground_color_axis
,foreground_color_border
,foreground_color_grid
,foreground_color_guide
,foreground_color_legend
,foreground_color_subplot
,foreground_color_text
,foreground_color_title
,framestyle
,grid
,gridalpha
,gridlinewidth
,group
,guide
,guidefont
,guidefontcolor
,guidefontfamily
,guidefontsize
,hover
,html_output_format
,inset_subplots
,label
,layout
,left_margin
,legend
,legendfont
,legendfontcolor
,legendfontfamily
,legendfontsize
,levels
,lims
,line_z
,linealpha
,linecolor
,linestyle
,linewidth
,link
,margin
,marker_z
,markeralpha
,markercolor
,markershape
,markersize
,markerstrokealpha
,markerstrokecolor
,markerstrokestyle
,markerstrokewidth
,normalize
,orientation
,polar
,primary
,projection
,quiver
,ribbon
,right_margin
,rotation
,scale
,series_annotations
,seriesalpha
,seriescolor
,seriestype
,show
,show_empty_bins
,size
,smooth
,subplot
,subplot_index
,tick_direction
,tickfont
,tickfontcolor
,tickfontfamily
,tickfontsize
,ticks
,title
,titlefontcolor
,titlefontfamily
,titlefonthalign
,titlefontsize
,titlefontvalign
,titlelocation
,top_margin
,weights
,window_title
,x
,xdiscrete_values
,xerror
,xflip
,xforeground_color_axis
,xforeground_color_border
,xforeground_color_grid
,xforeground_color_guide
,xforeground_color_text
,xgrid
,xgridalpha
,xgridlinewidth
,xguide
,xguidefontcolor
,xguidefontfamily
,xguidefontsize
,xlims
,xlink
,xrotation
,xscale
,xtick_direction
,xtickfontcolor
,xtickfontfamily
,xtickfontsize
,xticks
,y
,ydiscrete_values
,yerror
,yflip
,yforeground_color_axis
,yforeground_color_border
,yforeground_color_grid
,yforeground_color_guide
,yforeground_color_text
,ygrid
,ygridalpha
,ygridlinewidth
,yguide
,yguidefontcolor
,yguidefontfamily
,yguidefontsize
,ylims
,ylink
,yrotation
,yscale
,ytick_direction
,ytickfontcolor
,ytickfontfamily
,ytickfontsize
,yticks
,z
,zdiscrete_values
,zerror
,zflip
,zforeground_color_axis
,zforeground_color_border
,zforeground_color_grid
,zforeground_color_guide
,zforeground_color_text
,zgrid
,zgridalpha
,zgridlinewidth
,zguide
,zguidefontcolor
,zguidefontfamily
,zguidefontsize
,zlims
,zlink
,zrotation
,zscale
,ztick_direction
,ztickfontcolor
,ztickfontfamily
,ztickfontsize
,zticks
- Supported values for linetype:
:contour
,:heatmap
,:mesh3d
,:path
,:path3d
,:scatter
,:scatter3d
,:scattergl
,:shape
,:straightline
,:surface
,:wireframe
- Supported values for linestyle:
:auto
,:dash
,:dashdot
,:dot
,:solid
- Supported values for marker:
:auto
,:circle
,:cross
,:diamond
,:dtriangle
,:hexagon
,:hline
,:none
,:octagon
,:pentagon
,:rect
,:utriangle
,:vline
,:xcross
(Automatically generated: 2020-10-19T08:30:51.809)
This covers plotting the typical 2D and 3D plots in Julia with the Plots
package.
We will make use of some helper functions that will simplify plotting. These will be described in more detail in the following:
We will also use the ForwardDiff
for derivatives and use the 'prime' notation:
We will need to manipulate contours directly, so pull in the Contours
package, using import
to avoid name collisions and explicitly listing the methods we will use:
Finally, we need some features for vectors:
Parametrically described curves in space
Let $r(t)$ be a vector-valued function with values in $R^d$, $d$ being $2$ or $3$. A familiar example is the equation for a line that travels in the direction of $vec{v}$ and goes through the point $P$: $r(t) = P + t cdot vec{v}$. A parametric plot over $[a,b]$ is the collection of all points $r(t)$ for $a leq t leq b$.
In Plots
, parameterized curves can be plotted through two interfaces, here illustrated for $d=2$: plot(f1, f2, a, b)
or plot(xs, ys)
. The former is convenient for some cases, but typically we will have a function r(t)
which is vector-valued, as opposed to a vector of functions. As such, we only discuss the latter.
An example helps illustrate. Suppose $r(t) = langle sin(t), 2cos(t) rangle$ and the goal is to plot the full ellipse by plotting over $0 leq t leq 2pi$. As with plotting of curves, the goal would be to take many points between a
and b
and from there generate the $x$ values and $y$ values.
Let's see this with 5 points, the first and last being identical due to the curve:
Then we can create the $5$ points easily through broadcasting:
This returns a vector of points (stored as vectors). The plotting function wants two collections: the set of $x$ values for the points and the set of $y$ values. The data needs to be generated differently or reshaped. The function xs_ys
above takes data in this style and returns the desired format, returning a tuple with the $x$ values and $y$ values pulled out:
To plot this, we 'splat' the tuple so that plot
gets the arguments separately:
This basic plot is lacking, of course, as there are not enough points. Using more initially is a remedy. Rather than generate the ts
separately, xs_ys
has a simple frontend xs_ys(r, a, b)
which does this work itself:
Plotting a space curve in 3 dimensions
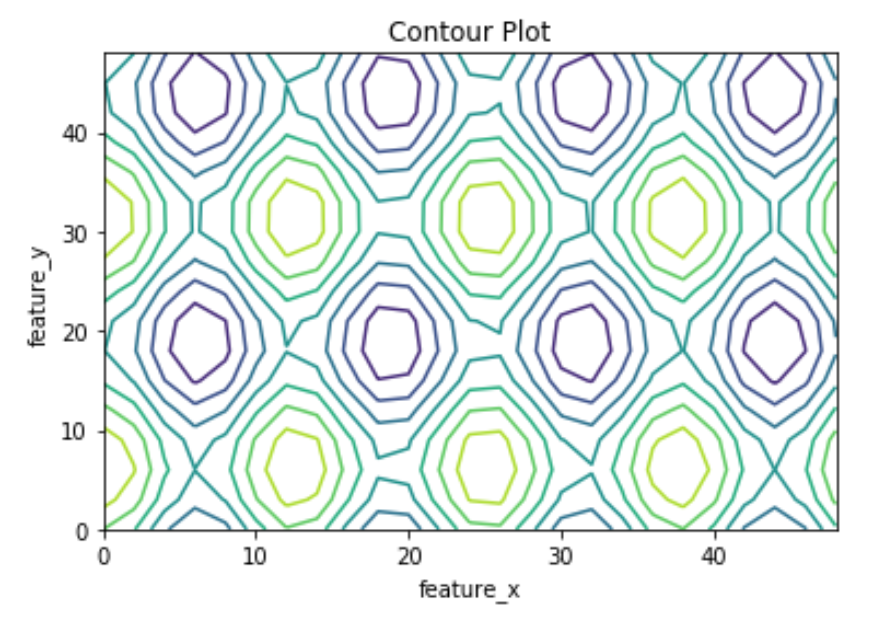
A parametrically described curve in 3D is similarly created. For example, a helix is described mathematically by $r(t) = langle sin(t), cos(t), t rangle$. Here we graph two turns:
Adding a vector
The tangent vector indicates the instantaneous direction one would travel were they walking along the space curve. We can add a tangent vector to the graph. The quiver!
function would be used to add a 2D vector, but Plots
does not currently have a 3D
analog. In addition, quiver!
has a somewhat cumbersome calling pattern when adding just one vector. So we have the arrow!
function defined above that uses quiver
for 2D arrows and a simple line for 3D arrows. As a vector incorporates magnitude and direction, but not a position, arrow!
needs both a point for the position and a vector.
Here is how we can visualize the tangent vector at a few points on the helix:
Setting a viewing angle for 3D plots
For 3D plots, the viewing angle can make the difference in visualizing the key features. In Plots
, some backends allow the viewing angle to be set with the mouse by clicking and dragging. Not all do. For such, the camera
argument is used, as in camera(azimuthal, elevation)
where the angles are given in degrees. If the $x$-$y$-$z$ coorinates are given, then elevation
or inclination, is the angle between the $z$ axis and the $x-y$ plane (so 90
is a top view) and azimuthal
is the angle in the $x-y$ plane from the $x$ axes.
Visualizing functions from $R^2 rightarrow R$
If a function $f: R^2 rightarrow R$ then a graph of $(x,y,f(x,y))$ can be represented in 3D. It will form a surface. Such graphs can be most simply made by specifying a set of $x$ values, a set of $y$ values and a function $f$, as with:
Rather than pass in a function, values can be passed in. Here they are generated with a list comprehension. The y
values are innermost to match the graphic when passing in a function object:
Remembering if the ys
or xs
go first in the above can be hard. Alternatively, broadcasting can be used. The command f.(xs,ys)
would return a vector, as the xs
and ys
match in shape–they are both column vectors. But the transpose of xs
looks like a row vector and ys
looks like a column vector, so broadcasting will create a matrix of values, as desired here:
This graph shows the tessalation algorithm. Here only the grid in the $x$-$y$ plane is just one cell:
A more accurate graph, can be seen here:
Contour plots
Returning to the
The contour plot of $f:R^2 rightarrow R$ draws level curves, $f(x,y)=c$, for different values of $c$ in the $x-y$ plane. They are produced in a similar manner as the surface plots:
The cross in the middle corresponds to $c=0$, as when $x=0$ or $y=0$ then $f(x,y)=0$.
Similarly, computed values for $f(x,y)$ can be passed in. Here we change the function:
The chosen levels can be specified by the user through the levels
argument, as in:
If only a single level is desired, as scalar value can be specified. For example, this next graphic shows the $0$-level of the [devil]](http://www-groups.dcs.st-and.ac.uk/~history/Curves/Devils.html)'s curve.
Contour plots are well known from the presence of contour lines on many maps. Contour lines indicate constant elevations. A peak is characterized by a series of nested closed paths. The following graph shows this for the peak at $(x,y)=(0,0)$.
Contour plots can be filled with colors through the contourf
function:
Combining surface plots and contour plots
In PyPlot
it is possible to add a contour lines to the surface, or projected onto an axis. Here is an example:
To replicate something similar, though not as satisfying, in Plots
we use the Contour
package.

There is no hidden line calculuation, in place we give the contour lines a transparency through the argument alpha=0.5
.
Gradient and surface plots
The surface plot of $f: R^2 rightarrow R$ plots $(x, y, f(x,y))$ as a surface. The gradient of $f$ is $langle partial f/partial x, partial f/partial yrangle$. It is a two-dimensional object indicating the direction at a point $(x,y)$ where the surface has the greatest ascent. Illurating the gradient and the surface on the same plot requires embedding the 2D gradient into the 3D surface. This can be done by adding a constant $z$ value to the gradient, such as $0$.
The tangent plane
Let $z = f(x,y)$ describe a surface, and $F(x,y,z) = f(x,y) - z$. The the gradient of $F$ at a point $p$ on the surface, $nabla F(p)$, will be normal to the surface and for a function, $f(p) + nabla f cdot (x-p)$ describes the tangent plane. We can visualize each, as follows:
From some viewing angles, the normal does not look perpendicular to the tangent plane. This is a quick verification for a randomly chosen point in the $x-y$ plane:
Parameterized surface plots
As illustrated, we can plot surfaces of the form $(x,y,f(x,y)$. However, not all surfaces are so readily described. For example, if $F(x,y,z)$ is a function from $R^3 rightarrow R$, then $F(x,y,z)=c$ is a surface of interest. For example, the sphere of radius one is a solution to $F(x,y,z)=1$ where $F(x,y,z) = x^2 + y^2 + z^2$.
Plotting such generally described surfaces is not so easy, but parameterized surfaces can be represented. For example, the sphere as a surface is not represented as a surface of a function, but can be represented in spherical coordinates as parameterized by two angles, essentially an 'azimuth' and and 'elevation', as used with the camera
argument.
Here we define functions that represent $(x,y,z)$ coordinates in terms of the corresponding spherical coordinates $(r, theta, phi)$.
We can parameterize the sphere by plotting values for $x$, $y$, and $z$ produced by a sequence of values for $theta$ and $phi$, holding $r=1$:
Plotting F(x,y, z) = c
Julia Bar Plot
There is no built in functionality in Plots
to create surface described by $F(x,y,z) = c$. An example of how to provide some such functionality for PyPlot
appears here. The following implements the same approach for Plots
.
To use it, we see what happens when a sphere if rendered:
The graphic is a bit hard to read. Showing only 1 set of slices with more levels can lead to an improvement:
This figure comes from a February 14, 2019 article in the New York Times. It shows an equation for a 'heart,' as the graphic will illustrate:
Vector fields
Consider a function $f: R^2 rightarrow R$. The gradient function, $nabla f$ is a function from $R^2$ into $R^2$. Such functions can be visualized in terms of a vector field. For each point, $p$, the gradient (or a scaled version) is indicated by anchoring it at $p$. This is typically done for a grid of values. In the code below, we scale the size of each vector so that they stay within the grid.
We see the use of quiver
to add vectors in 2D. The ps
need to be specified as xs, ys
, hence the splatting, whereas the quiver
argument expects a tuple of vectors, hence no splatting. Calling quiver
this way, as opposed to adding individual lines through arrow
, say, is much more efficient.
The scale used is simply computed. The points are spaced evenly with $4/10$ units between them. The longest vector will have this length, so will not be able to leave the cell, hence the vectors won't overlap, as would happen with this example otherwise.
Julia Plots 3d
One last comment, in place of the comprehension to form the vs
, broadcasting, as with vs = ForwardDiff.gradient.(f, ps)
, could be used.